AI Foundations for Retail Executives: Key Concepts Every Business Leader Should Know
Our AI Ambassador and Senior Consultant Peter Beck and our CEO Gabor Tozser introduce main AI concepts
AI for retail executives is no longer a futuristic concept—it’s a present-day reality transforming the industry. Artificial Intelligence (AI) and Machine Learning (ML) are now integral to our daily lives, with these technologies powering everything from personalized recommendations on streaming platforms to self-driving cars. AI is also transforming industries worldwide, and retail is no exception. From personalized customer experiences to automated inventory management, AI is reshaping how businesses operate and compete. However, many IT stakeholders struggle to differentiate between AI myths and reality. Understanding AI fundamentals is the first step toward leveraging its full potential.
This article will introduce key AI concepts every IT leader should know. By grasping these basics, decision-makers can build a solid foundation for future AI-driven strategies.
What is Artificial Intelligence
AI enables machines to simulate human intelligence, allowing software systems to process information, learn from experience, and perform tasks that typically require human cognition. There are two main types of AI:
- Narrow AI – Designed for specific tasks like chatbots, recommendation engines, or virtual assistants (e.g., Siri, Alexa).
- General AI – Theoretically capable of performing any intellectual task that a human can, though this remains a distant possibility.
Understanding AI is increasingly important as it transforms multiple industries, including healthcare, finance, education, retail, and entertainment. For business leaders, a foundational knowledge of AI helps drive informed decision-making and unlock new competitive advantages.
Key AI Foundations Every Retail Executive Should Know
AI Agent
An AI agent is a system that perceives its environment and takes actions to achieve a goal. For example, a self-learning chatbot in customer service acts as an agent by responding to queries based on learned patterns.
Prompt
A prompt is the input given to an AI model to generate a response. Users provide text-based prompts to AI-powered assistants to receive accurate and context-aware replies.
AI Model
The AI model is the core engine of an AI system. It processes data, identifies patterns, and generates outputs. AI models can be trained for various tasks, including image recognition, fraud detection, and language translation.
Training Data
AI models require large datasets to learn and make accurate predictions. The quality of training data directly impacts AI performance. Retail AI applications, such as demand forecasting and personalized marketing, rely heavily on high-quality data.
Inference
Inference is the process where a trained AI model applies its learned knowledge to new, unseen data. For instance, when a recommendation engine suggests products based on a customer’s browsing history, it is performing inference.
Machine Learning (ML)
ML is a subset of AI focused on developing algorithms that enable machines to learn from data without explicit programming. Think of ML as teaching a machine how to bake cookies:
- Ingredients (Data): The raw materials needed for learning.
- Recipe (Algorithm): The set of instructions for combining ingredients.
- Oven (Model Training): The process where raw ingredients transform into cookies.
- Tasting (Evaluation): Assessing whether the cookies meet expectations.
Neural Networks & Deep Learning
Neural networks are computational models inspired by the structure of the human brain. They consist of interconnected layers of nodes (neurons) that process data hierarchically. Deep learning refers to neural networks with multiple layers, enabling them to learn complex patterns in data.
Analogy: Think of a neural network as an assembly line in a factory:
- Each worker (neuron) performs a specific task.
- Workers are organized into teams (layers), each specializing in different aspects of production.
- Information flows along the assembly line until the final product is ready.
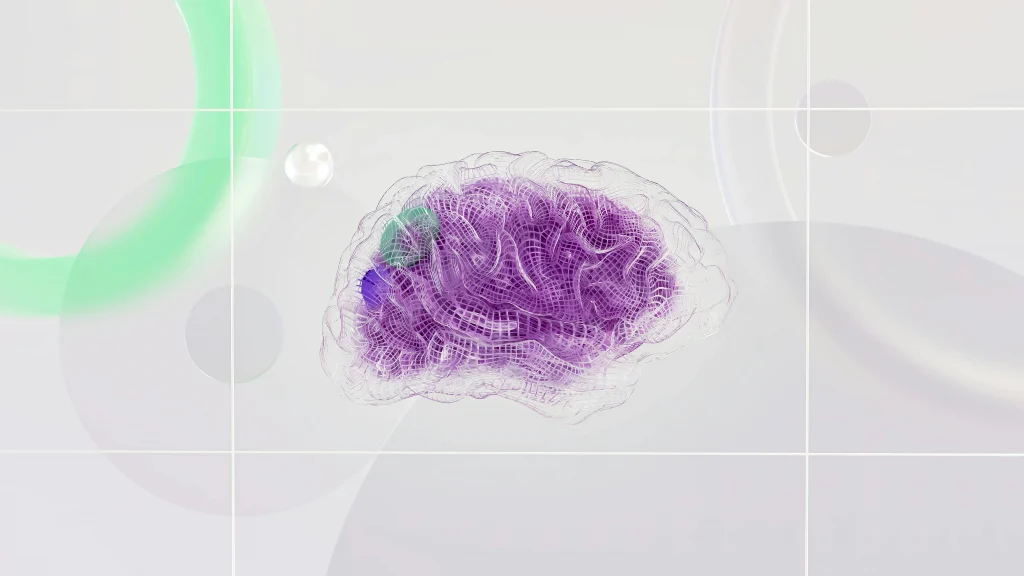
How AI Works
AI operates in a cycle with three key phases:
- Training: AI models learn from vast datasets to recognize patterns and make predictions.
- Fine-Tuning: Once trained, models are refined and optimized for specific business use cases.
- Real-World Application: The AI model is deployed to perform real-time tasks, such as fraud detection or automated customer service.
AI models continuously improve over time through feedback loops and additional training data.
Debunking Common AI Myths
Myth: AI will replace all jobs.
Reality: AI enhances human decision-making by automating repetitive tasks, allowing employees to focus on higher-value activities.
Myth: AI can think like a human.
Reality: AI operates based on mathematical models and data, lacking consciousness or emotional intelligence.
Myth: AI is always accurate.
Reality: AI can be biased or inaccurate if trained on flawed data, making ethical considerations and human oversight essential.
Why AI Matters for Retail Executives
Retail businesses can leverage AI to drive efficiency and innovation. Key applications include:
AI-Driven Decision Making
AI analyzes data to optimize pricing, inventory management, and customer segmentation, helping retailers maximize profitability.
Personalized Customer Experiences
AI tailors product recommendations and marketing messages, enhancing customer satisfaction and boosting sales.
Operational Efficiency
AI automates supply chain processes, reducing costs, improving logistics, and ensuring demand-driven inventory management.
As AI adoption grows, IT leaders must understand its fundamentals to make informed decisions about implementation, scalability, and risk mitigation.
Conclusion
AI is not just a buzzword—it is a critical tool for modern business success. Understanding its foundational concepts enables IT stakeholders to evaluate AI opportunities and mitigate risks effectively.
Why Retail Executives Should Develop AI Strategies Now
AI is already reshaping the retail landscape. IT leaders who develop AI strategies today will be better positioned to drive innovation, reduce operational inefficiencies, and stay ahead of the competition.
The next article in this series will explore practical AI applications in retail and how companies can integrate AI into their business processes.
Stay tuned for the next installment on real-world AI use cases and strategies for successful AI adoption in retail
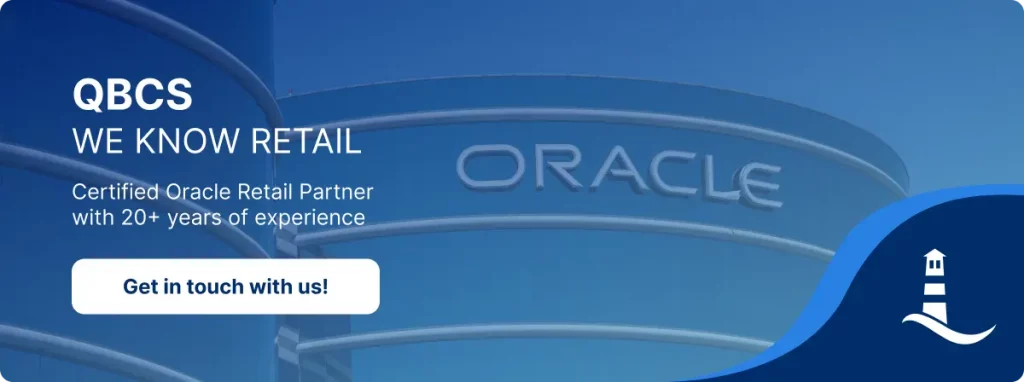